Univ.-Prof. Dr. Gasper Tkacik
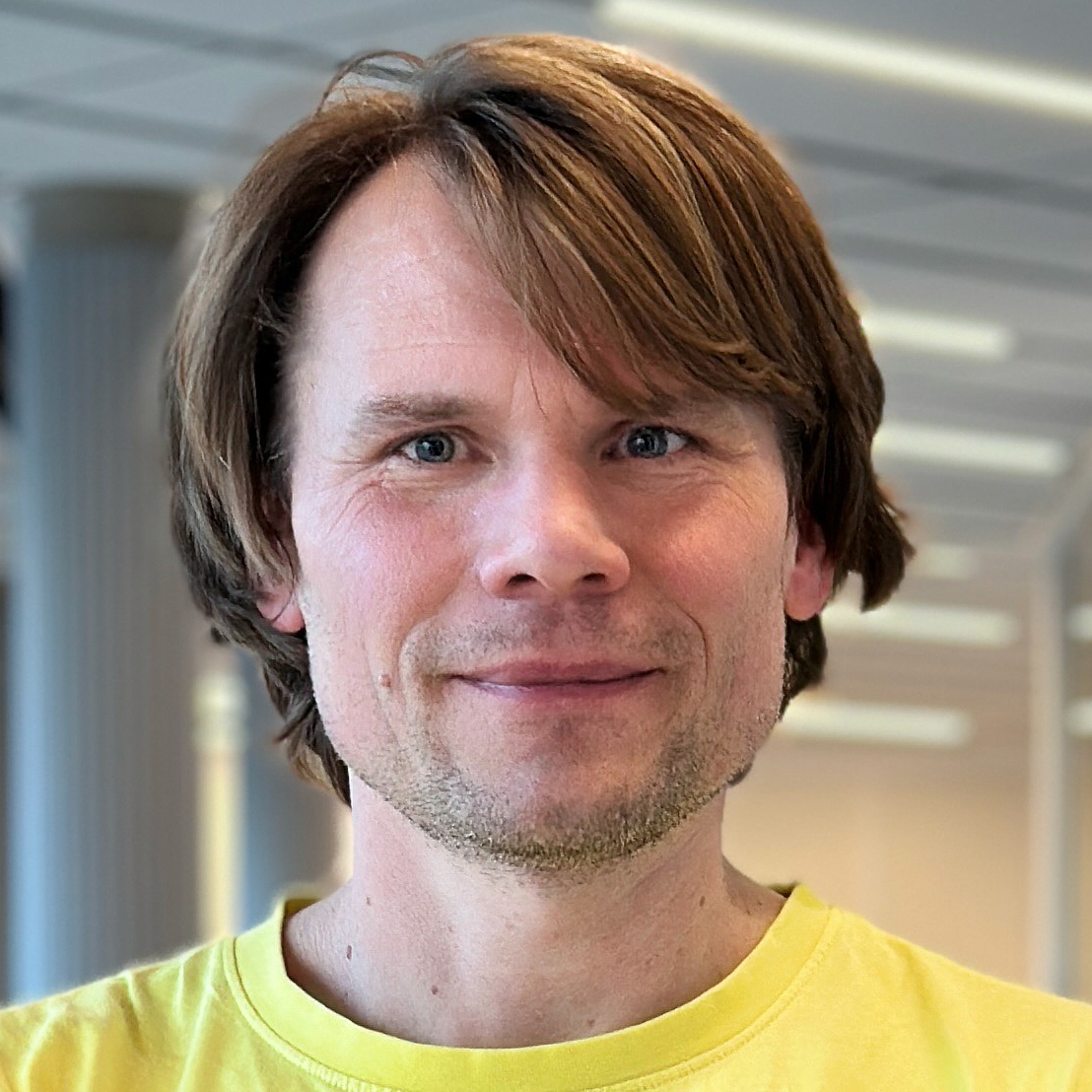
The primary aim of our group is a theoretical understanding of how reliable information transmission, computation, and decision-making can arise in realistic networks (neural, cell signaling, gene regulation, etc.) that develop from typically noisy, delayed, and heterogeneous components. Our neuroscience focus is on theories of neural coding that originated in sensory periphery (efficient, sparse, predictive, etc. coding) which we try to extend into central neural processing. Even more specifically, recently we have been focusing on two directions:
- Normative theories applied to efficient stimulus encoding in task-driven, feedbacked systems, which are able to, ab initio, predict attentional modulation (Mlynarski & Tkacik, 2022).
- The interplay between stimulus- and spontaneous large-scale brain activity, its functional implications, and relation to brain criticality (Lombardi et al, 2023).
Focal points of interest
At the level of abstraction we work at, models do not discriminate between different types of inhibitory cells; moreover, most computational theories of coding (and, by consequence, most machine learning architectures) completely ignore the E/I differences. Our goal is to address these gaps in theory and modeling. With respect to the two foci above, we propose to:
- Specialize efficient coding models by explicitly including feedback-mediated inhibition that can modulate the gain of individual primary sensory neurons, or mediate lateral interactions between such neurons, for instance, to model the neural substrate of primary visual cortex which would receive top-down signals that change individual neuron receptive fields;
- Show the necessity for inhibition to explain large scale spontaneous brain activity with oscillations and critical avalanches. These directions are driven by theoretical (our group) and experimental (Joesch lab, with whom we collaborate) considerations. In collaboration with the Joesch lab, we are building minimalistic, non-equilibrium Ising-model-inspired networks that, via local excitation and longer-range inhibition, generate patterns of spontaneous activity that the Joesch lab records in freely behaving mice in the superior colliculus. Within this collaboration, the next step would be to pursue mechanistic questions about inhibitory neuron subtypes and links to behavioral performance.
Technical proficiency and instrumentation
We bring together statistical and biological physics, data analysis, machine learning, evolution, and neuroscience; we dedicate ~50% of our time to theory and ~50% to data-driven and collaborative projects. Our contributions are considered to be at the forefront of the application of information-theoretic methods and quantification to biological networks. In neuroscience, we have contributed significantly to the inference of large-scale data-driven maximum-entropy models (i.e., “inverse Ising” or maxent models), that can capture neural population activity – excitatory as well as inhibitory – at the detailed single-spike level and match the data exactly in various measured statistics. We have also advanced normative (optimal) models of population codes, and developed rigorous statistical methodology to test the predictions of high-dimensional optimality theories against experimental data (Mlynarski et al, 2021).
Aspirations for the next 5 years
- We will advance the theory of noisy & feedbacked efficient neural coding by combining information with control theory, a recent new focus in our group, always staying grounded in the observations of our experimental colleagues in the Joesch lab.
- We will work with both Joesch and Csicsvari labs to understand the role of inhibition in, and the functional implications of, spontaneous neural activity (closeness to criticality, inhibition-mediated spatio-temporal activation patterns etc.) for task performance as well as memory and learning, both in superior colliculus and in hippocampus (Nardin et al, 2023).
References
- Nardin M, Csicsvari J, Tkacik G, Savin C (2023) The structure of hippocampal CA1 interactions optimizes spatial coding across experience. Journal of Neuroscience 43, 8140–8156.
- Lombardi F, Pepic S, Shriki O, Tkacik G, De Martino D (2023) Statistical modeling of adaptive neural networks explains coexistence of avalanches and oscillations in resting human brain. Nature Computational Science 3, 254263.
- Mlynarski WF, Tkacik G (2022) Efficient coding theory of dynamic attentional modulation. PLOS Biology 20, e2001889.
- Mlynarski WF, Hledik M, Sokolowski TR, Tkacik G (2021) Statistical analysis and optimality of neural systems. Neuron 109, 1–15.