Univ.-Prof. Dr. Tim Vogels
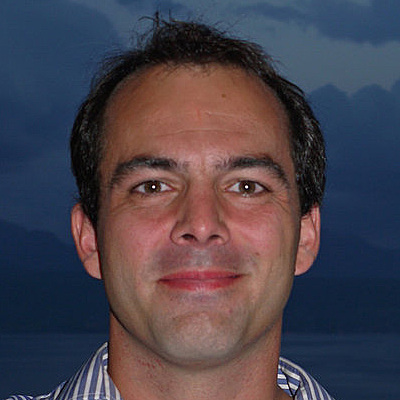
The Vogels lab aspires to understand the interplay of neural network dynamics and synaptic plasticity at excitatory and – in particular – inhibitory synapses. The lab interest focuses on the rules that dictate how cortical activity can shape networks so as to form reliable sensory perceptions, stable memories, and motor outputs. From its opening, the lab has been aiming to build models of neurons and neuronal networks that distill and re-articulate our current knowledge of how nervous systems compute at a mechanistic, implementational level of understanding. Most recent work argues for a much tighter partnership of excitatory and inhibitory synapses during learning than previously thought, and investigates the link of neural metabolism and activity, providing a novel perspective of the usefulness of ubiquitously observed spontaneous activity.
Focal points of interest
Through Vogels’s early work on cortical network models it became clear to him that GABA neurons, despite fewer in numbers compared to pyramidal neurons, play a critical role in stabilizing and controlling cortical network dynamics. They maintain homeostasis within the network and influence the computational processes, suggesting a complex hierarchy of control mechanisms with a number of specialized functions, essential for the intricate balance and functionality of the cortex. With this realization, the question of how these neurons learn to exert control so seemingly precisely rose. This question led to long standing personal interest and concurrently a lab focus on inhibitory synaptic plasticity. Vogels believes that understanding GABAergic plasticity rules is essential for solving tuning problems in neural networks, as excitatory synapses cannot function effectively without the interplay of inhibitory synapses. Moreover, inhibitory synaptic function is, at a first approximation, much easier to articulate due to its clear homeostatic target. Finally, in addition to being crucial for illuminating the organisation of neural dynamics, understanding GABA synaptic plasticity also has large implications for artificial neural networks in which the learning rules for complex function are still as poorly understood as in biology, and where inhibitory mechanisms could contribute to challenging tuning issues.
Technical proficiency and instrumentation
The lab implements biologically plausible, spiking network models in a vast array of model frameworks ranging from standard models in the Brian framework, to highly specialized models directly in Python or C++. Recently we have begun to include modern methods of machine learning to search for biological realistic plasticity rules with large computational effort. In fewer words, the lab is specialized in Neurotheory, Computational Neuroscience and NeuroAI.
Aspirations for the next 5 years
For the next five years, the Vogels group will work on understanding the interaction of 4 or more plasticity rules in a single neural network model, to produce a working hypothesis of how brains can learn and maintain stability at the same time. As such, the lab will continue to utilize the ever growing power of machine learning methods to investigate synaptic plasticity rules directly, and design experimental protocols to test them with our collaborators.
References
- Agnes EJ , Vogels TP (2024) Co-dependent excitatory and inhibitory plasticity accounts for quick, stable and long-lasting memories in biological networks. Nature Neuroscience 27, 964–974.
- Confavreux B, Ramesh P, Goncalves PJ, Macke JH, Vogels TP (2023) Meta-learning families of plasticity rules in recurrent spiking networks using simulation-based inference. Advances in Neural Information Processing Systems 36, 13545–13558.
- Confavreux B, Zenke F, Agnes E, Lillicrap T, Vogels TP (2020) A meta-learning approach to (re)discover plasticity rules that carve a desired function into a neural network. Advances in Neural Information Processing Systems 33, 16398–16408.